Copyright notice:
The material is presented to ensure timely dissemination
of scholarly and technical work. Copyright and all rights
therein are retained by authors or by other copyright holders.
All persons copying this information are expected to
adhere to the terms and constraints invoked by each
copyright. In most cases, these works may not be
reposted without the explicit permission of the
copyright holder.
If you want a paper that is not available here,
or if you have any problems accessing these documents,
e-mail me.
k-support norm regularization to fMRI analysis.
An exploration of various sparse regularization techniques for analyzing
fMRI data, such as the L1 norm (often called LASSO in the context of a
squared loss function), Elastic Net, and k-support norm. Employing sparsity
regularization allows us to handle the curse of dimensionality, a problem
commonly found in fMRI analysis. In this work we consider sparse regularization
in both the regression and classification settings. We perform experiments
on fMRI scans from cocaine-addicted as well as healthy control subjects.
Publications
-
Predictive sparse modeling of fMRI data for improved
classication, regression, and visualization using
the k-support norm.
Belilovsky E., Gkirtzou K. Misyrlis M., Konova A.B., Honorio J., Alia-Klein N., Goldstein R., Samaras D., and Blaschko M.
Computerized Medical Imaging and Graphics, 2015
[pdf] [bibtex] [doi] [code] -
fMRI Analysis of Cocaine Addiction Using
k-support Sparsity.
Gkirtzou Katerina, Honorio Jean, Samaras Dimitris, Goldstein Rita and Blaschko B. Matthew
International Symposium on Biomedical Imaging (ISBI), 2013.
[pdf] [bibtex] [doi] -
Sparsity regularization and graph-based
representation in medical imaging
Gkirtzou Katerina
PhD Thesis, Ecole Centrale de Paris, 2013.
[pdf] [bibtex] [presentation]
Code
The code is provided under the GNU GPL license. It does not come with any warranty of any kind.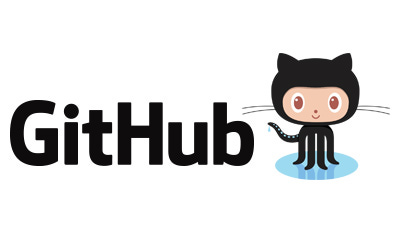